AI and cybersecurity overview
Your organization is likely facing more cyber threats than ever before, but AI is changing how you can detect these threats, reduce risks, and keep pace with your digital operations. Artificial intelligence now plays a significant role in threat detection, response, automation, and vulnerability management.
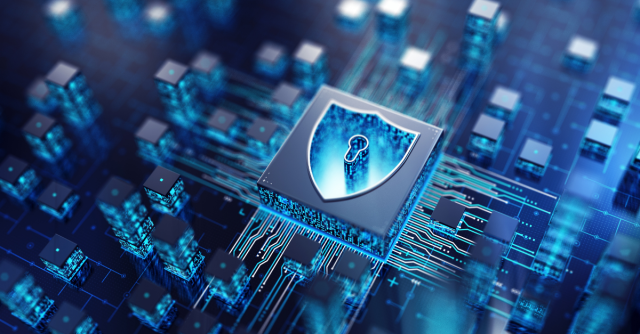
When used effectively, AI helps your security team scale efforts without adding staff. But it also introduces new risks, especially as cybercriminals use the same technology to create smarter attacks. As these tools evolve, questions about ethics, trust, and control become increasingly important.
This blog breaks down how AI influences cybersecurity today, what technologies are shaping the future, and how cybersecurity services from Flexential support smarter, more secure infrastructure.
What is artificial intelligence (AI) in cybersecurity?
When we talk about AI in cybersecurity, we mean smart systems that can spot and respond to threats with minimal human input. These technologies work by finding patterns and flagging unusual activity much faster than traditional tools.
AI processes massive amounts of data from networks, endpoints, and cloud environments to identify risks in real time. This includes detecting malware, phishing attempts, insider threats, and other suspicious behavior—often before a breach happens.
Unlike traditional tools with fixed rules, AI adapts to new information. This makes AI particularly valuable as threats constantly change and become harder to spot with conventional methods.
Your cybersecurity teams can use AI to complement human expertise, not replace it. When applied correctly, AI reduces false positives, improves response times, and scales security operations beyond what manual processes can achieve.
How is generative AI used in cybersecurity?
Generative AI affects both sides of cybersecurity, helping your defenders strengthen systems while giving attackers new tools to exploit vulnerabilities.
For defense, you can use generative AI to simulate realistic attack scenarios, create threat intelligence reports, and automate detection rules. Security teams train models to produce synthetic data sets mimicking complex attack patterns, which helps test and improve detection systems.
It also supports communication. Generative models can draft security documentation, summarize incident reports, or translate technical details into language non-technical stakeholders can quickly understand.
But there are risks. Bad actors use this same technology to create more convincing phishing emails, malware code, and deepfake content. This dual-use challenge makes it important to approach generative AI with caution and clear governance.
The impact and evolution of AI on cybersecurity
AI has moved from an experimental concept to a core component of modern cybersecurity strategies. Early applications focused on automation, such as reducing alert response time and handling repetitive tasks. As the technology advanced, its role expanded to active threat detection, behavior analysis, and predictive modeling.
Machine learning models now help identify previously unknown threats by detecting anomalies in user behavior, network traffic, and system activity. These tools allow your security team to find patterns that traditional rule-based systems would miss.
At the same time, the threat landscape has grown more complex. Attackers now adopt AI to improve their techniques, automate reconnaissance, and avoid detection. This raises the stakes, forcing defenders to innovate faster and use AI not just for efficiency, but for resilience.
The evolution of AI and data security is ongoing. As your organization adopts new architectures and cloud services, AI becomes essential in helping cybersecurity systems scale and adapt.
Use cases for AI in cybersecurity
You can apply AI across many security functions today. Some of the most effective uses include:
- Identity and access management (IAM): Spot suspicious logins and unusual access behavior in real time
- Endpoint security: Monitor devices for signs of compromise and respond faster than traditional antivirus tools
- Cloud security: Analyze cloud traffic patterns to detect misconfigurations, unauthorized access, and policy violations
- Cyberthreat detection: Use machine learning to identify malware, ransomware, and other threats based on behavior—not just signatures
- Information protection: Classify sensitive data and enforce policies to prevent leaks or unauthorized sharing
- Incident response: Automate investigation steps, connect related alerts, and suggest fixes based on previous incidents
- Security analytics: Process large volumes of data to find anomalies and identify long-term trends
These applications show how AI is transforming cybersecurity from a reactive model to a more predictive, proactive strategy.
Key AI technologies used in cybersecurity
Several core AI technologies drive advancements in cybersecurity. Each plays a different role in helping your team identify threats, automate responses, and make smarter decisions under pressure.
Machine learning and its applications
Machine learning (ML) forms the foundation of most AI-based cybersecurity tools. These models learn from historical data to detect patterns, predict threats, and classify behavior as normal or suspicious. Supervised ML can identify known threats, while unsupervised models surface unknown risks by flagging outliers. Common applications include intrusion detection systems, malware classification, and real-time risk scoring.
Natural language processing in threat detection
Natural language processing (NLP) allows AI systems to understand and analyze human language. In cybersecurity, NLP helps monitor communication channels for phishing attempts, extract insights from threat intelligence reports, and process log files or security alerts written in plain text. It's also used in chatbots and virtual assistants that support security operations by answering questions and guiding incident response.
Neural networks and anomaly detection
Neural networks are AI systems modeled loosely after the human brain. They're particularly useful for detecting anomalies that may indicate a security incident, such as unusual login patterns or data transfer behavior. Deep neural networks can analyze large datasets across multiple variables to identify subtle signals that would be missed by simpler models. This makes them valuable for high-stakes environments where early detection is critical.
Together, these technologies help build smarter, faster, and more adaptive cybersecurity defenses, especially when integrated into a broader security strategy.
CISA’s strategic roadmap for artificial intelligence
The Cybersecurity and Infrastructure Security Agency (CISA) has taken a proactive role in shaping how AI is developed and used within critical security systems. In 2024, the agency released its AI roadmap, outlining key priorities for safe and effective integration of artificial intelligence in both public and private sectors.
CISA’s strategy focuses on five core areas:
- Ensuring secure AI adoption across federal systems and critical infrastructure
- Protecting against malicious use of AI, including deepfakes and automated cyberattacks
- Promoting transparency and accountability in AI development and deployment
- Strengthening partnerships with industry, academia, and international stakeholders
- Building AI expertise through workforce development and upskilling programs
This roadmap sets a framework for responsible AI use that balances innovation with risk management. For organizations building AI into their cybersecurity programs, aligning with CISA’s principles offers a way to stay ahead of regulations and avoid common implementation pitfalls.
Key benefits of AI in cybersecurity
If your security team is stretched thin with growing threats and constant alerts, AI offers clear advantages. These technologies can strengthen your defenses without adding complexity—if you implement them thoughtfully.
Improved threat detection and response times
AI processes large volumes of security data in real time, allowing your team to spot threats faster than traditional tools. Machine learning models detect anomalies and malicious behavior with greater precision, reducing false positives and helping analysts focus on real issues. This speed is critical for limiting damage during an active breach or stopping attacks before they escalate.
Automation of repetitive security tasks
Security teams often spend hours handling tasks like log analysis, alert triage, and incident documentation. AI automates many of these processes, freeing up time for higher-value work. Automated playbooks can respond to known threats, escalate critical issues, and even isolate compromised systems—without waiting for manual input.
Enhanced data analysis and prediction
AI models find patterns and trends that would take humans much longer to uncover. In cybersecurity, this means identifying early indicators of compromise, predicting where new threats might emerge, and helping your team proactively adjust defenses. Over time, these insights support more resilient systems and better decision-making.
Challenges and risks of AI in cybersecurity
While AI brings clear advantages to your cybersecurity program, it also introduces new risks that you must consider. These risks range from technical limitations to ethical concerns and the growing threat of AI being used by attackers.
Potential for AI-driven cyber attacks
AI is not exclusive to defenders; cybercriminals actively use it to launch more sophisticated attacks. Generative AI makes it easier to create convincing phishing emails, spoofed messages, and malware variants that bypass traditional filters. Attackers also use AI for reconnaissance, scanning systems for vulnerabilities and identifying weak points more efficiently than before.
To better understand this threat, watch the Flexential webinar on hackers using AI and cybersecurity, which explores real examples and strategies for defense.
Ethical and privacy concerns
AI systems are only as good as the data they're trained on, and how that data is used matters. There are growing concerns about surveillance, consent, and the unintended consequences of automating decisions that impact people. If your AI systems are not properly governed, they can reinforce bias, violate privacy policies, or make enforcement decisions without transparency or accountability.
Dependence on data quality and quantity
AI models require large, high-quality datasets to function effectively. Poor data quality—such as incomplete logs, outdated threat intelligence, or biased inputs—can lead to incorrect results. This creates a false sense of security and may cause your team to overlook real threats or misclassify benign behavior. Maintaining a reliable data pipeline is essential for keeping AI systems accurate and useful.
Implementing AI in cybersecurity strategies
Adding AI to your cybersecurity strategy isn't just about installing new tools; it requires alignment with existing frameworks, investment in skills development, and a commitment to responsible use. For AI to be effective, it needs to work within the broader context of your organization's goals, policies, and security posture.
Integrating AI with existing security frameworks
Your AI solutions should complement, not replace, the technologies and processes already in place. That means integrating AI into SIEM systems, incident response workflows, and governance structures so that insights from AI can be acted on quickly and consistently. Our paper on accelerating your cybersecurity maturity journey outlines how you can evolve your program to include automation and intelligence without creating gaps or silos.
Training and development for cybersecurity professionals
AI tools are only as effective as the teams using them. That's why it's critical to provide training that helps your security professionals understand how AI works, where it fits, and how to validate its outputs. Upskilling initiatives should focus on core concepts like machine learning, data analysis, and responsible AI use, alongside practical application in threat detection and response.
Best practices for AI-driven security solutions
To use AI effectively, your organization should:
- Start with clear goals and metrics for success
- Choose tools that offer transparency and explainability
- Regularly test and audit AI models for accuracy and bias
- Align AI use with regulatory and compliance requirements
- Build cross-functional teams that include data scientists, engineers, and security analysts
By taking a thoughtful and structured approach, organizations can get real value from AI without adding unnecessary risk.
The future of AI and cybersecurity
AI's role in cybersecurity continues to grow, and so does the need for thoughtful leadership, policy, and cross-industry collaboration. The next phase won't just be about better tools, but better coordination between people, data, and intelligent systems.
Trends shaping AI’s role in cyber defense
Organizations increasingly adopt AI to manage risk across hybrid environments, cloud systems, and data centers. AI is now embedded in everything from zero trust architectures to automated threat intelligence. According to a recent AI and cybersecurity survey, enterprise IT leaders are embracing AI not just to keep up, but to get ahead of evolving threats.
Another important shift is the focus on infrastructure—particularly how AI workloads affect performance, cost, and energy use. Flexential explores this further in our blog on AI data center trends.
Predictions for AI-driven security innovations
Expect to see more AI systems that combine different models—like machine learning, natural language processing, and graph analytics—to create multi-layered defense strategies. Vendors are also beginning to incorporate AI into identity verification, behavioral biometrics, and zero-day exploit detection.
At the same time, attackers will likely continue refining their use of AI, especially for social engineering and ransomware delivery. Your organization will need to keep evolving defenses, making AI part of a broader threat intelligence and resilience strategy. Read more about practical steps in counter ransomware threats.
Building a collaborative future between AI developers and cybersecurity experts
Security isn't just a technical challenge—it's a team effort. To get the most from AI, your cybersecurity leaders will need to work closely with developers, data scientists, compliance teams, and even ethicists. This collaboration ensures that tools are tested, explainable, and aligned with company policies and user expectations.
As tools become more complex, this kind of shared responsibility will be critical to keeping systems trustworthy and effective, especially as regulations catch up with the pace of innovation.
Key takeaways and frequently asked questions
AI is reshaping cybersecurity, from how threats are detected and analyzed to how teams respond and adapt. While the benefits are significant, so are the risks. Success depends on using AI in ways that are informed, strategic, and supported by the right expertise, frameworks, and oversight.
Flexential brings this expertise to every client engagement, helping organizations strengthen their security posture with scalable, intelligent solutions. Explore more trends on AI cybersecurity and stay ahead of what’s next.
Explore more
Looking to strengthen your security strategy with AI-driven solutions?
- Watch our webinar on how hackers are using AI and how to stop them
- Download the paper on accelerating your cybersecurity maturity journey
- Or contact our team to talk about your goals and how we can help
FAQs
How is AI used in cybersecurity?
AI is constantly scanning your systems for unusual patterns that might signal trouble, processing mountains of data from across your network in seconds. Where traditional tools can only spot known threats (like recognizing a face they've seen before), AI can identify suspicious behavior even from brand-new threats it's never encountered.
Will cybersecurity be replaced with AI?
Not a chance. What's happening instead is a powerful partnership. AI handles the heavy lifting—processing data, spotting patterns, flagging anomalies—while your human experts bring critical thinking, judgment, and creativity to the table. It's like having both a sophisticated alarm system AND experienced security guards. The machines alert, the humans evaluate and decide.
What are some examples of AI in cybersecurity?
Examples include intrusion detection systems using machine learning, phishing detection with NLP, and automated malware analysis tools that adapt based on threat behavior.
Why should businesses use AI in cybersecurity?
Threats are multiplying faster than security teams can grow, and AI helps you scale your defenses without an equivalent scaling of headcount. It's particularly valuable when your team is drowning in alerts or when you need eyes on multiple environments simultaneously. Think of it as having digital security analysts working alongside your team, handling the routine so your experts can focus on what matters.
Can AI completely replace human cybersecurity experts?
The short answer: no. The longer answer: definitely not. AI can process data and spot patterns at incredible speed, but it can't understand context like humans do. It can't grasp the full implications of a breach, negotiate with stakeholders, or make ethical judgments in gray areas. AI is your security team's powerful tool, not their replacement.
Is AI adoption only for large corporations?
That might have been true five years ago, but not anymore. Today's managed security services bring AI capabilities within reach of smaller organizations. You don't need a data science team or massive infr astructure investments to benefit. Many platforms now offer AI security features out-of-the-box, making advanced protection accessible regardless of your company size.
How can organizations ensure AI ethics in cybersecurity?
Start by treating AI like any powerful tool, with clear policies about who can use it and how. Create a diverse team to oversee AI implementation, including voices from security, legal, privacy, and business units. Regularly test your AI systems not just for effectiveness but for bias and fairness. Remember: an AI is only as ethical as the humans who design, deploy, and govern it.
What is the AI strategy for cybersecurity?
Start with your security goals and work backward to where AI fits. Identify specific challenges where AI can make the biggest difference, like alert overload or monitoring cloud environments. Then integrate AI tools with your existing systems, train your team to work with them effectively, and continuously measure results. It's evolution, not revolution.
Are there courses offered for AI and cybersecurity?
Yes, and they're becoming increasingly practical. Beyond general AI and security foundations, look for training that addresses real-world scenarios: How do you interpret AI security alerts? When should you override automated recommendations? What questions should you ask vendors about their AI models? The most valuable courses teach you to be an informed AI user, not just explain how the technology works.