Catching the AI waves
Like any advanced technology, it starts in a lab inspired by a group of advanced thinkers, solving problems that exist today with impacts well into the future. The birth of artificial intelligence, “AI,” isn’t any different.
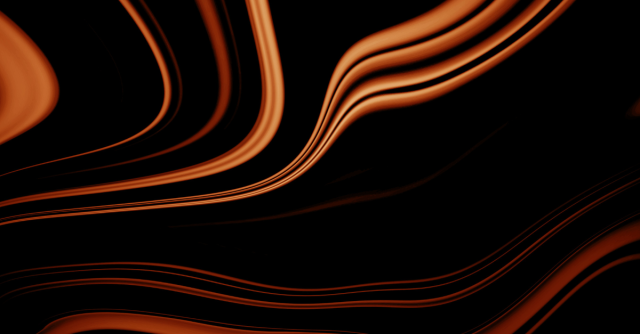
From concept to hybrid reality
The first recorded use of “AI” as a term or concept was coined by a group of scientists brought together in 1956 at Dartmouth College. This group, inspired by earlier concepts from Alan Turing and Herbert Simon, envisioned how human-like intelligence and interaction might be managed electronically. This was near the dawn of scalable computing machines with limited capabilities, taking up entire buildings to do simple math. Now we harness that power (and beyond!) in our smartwatch.
As AI has continued to develop, it’s been powered by fast processing, high-performance computing and networks, and software to make it easier to use. Machine learning is now commonplace on many platforms, helping detect patterns to increase the visibility of problems, discovering solutions, and assisting humans in many applications.
The Four Waves of AI defined
In 2021, as part of their effort to identify the AI super use case, BGV (Benhamou Global Ventures, a leading VC firm) defined what they called the “Four Waves of AI”. Let’s look at where we are in relation to this concept and how we might right the tide into the future.
[Image credit: Deep Recommendation as the AI Super Use Case - BGV]
Wave 1: Research ecosystem
The first wave started within the research ecosystem, steeped in computer science, the foundation of where AI evolved in the first place. Over the years, AI has been experimentally used to emulate human thought, play chess, help diagnose patients, play Jeopardy! or analyze targets for war. It was highly specialized, expensive, and used in unique situations. Early on, an IBM scientist estimated that the platform that won Jeopardy! was really good at trivia but had a second-grade reading level at best. AI was rudimentary in its general results, most applicable to solving specific problems, like chess, that can be easily proved mathematically.
Wave 2: Cloud AI
In the second wave of AI, access to high-performance compute (HPC), software to utilize it, and the broad expanse of network-accessible data has been widely enabled by public cloud providers like Amazon, Microsoft, and Google. Advanced graphics processing units (GPUs) are readily available, shrinking the time to value and results in every new design. AI is computationally (math) heavy; the software reduces problems into math equations that GPUs can help process.
In this phase, the training, or what could be called the art and science of getting the results right, is key. It’s estimated that OpenAI’s ChatGPT 3 used 10,000 GPUs, took approximately 15 days to train, and consumed over 1GW of electricity! Over 175 billion objects (data) were used to create the ChatGPT3 generative AI platform. All that gets translated to predictive math—to produce query results, learning along the way. This second wave enabled generative AI to the masses via a browser. This is a seminal moment like the Internet being accessed via Mosaic instead of an email address or Gopher.
Wave 3: Mainstream enterprise
We are at a big inflection point for AI, entering the mainstream wave where “the revolution will be hybridized.” According to many, 2023 will be known as the “iPhone” year for AI. Enterprises are starting to evaluate how AI can be used to accelerate business and reduce costs. Non-profits are looking at how to deepen engagement with their members and partners. Some organizations are even taking somewhat of a “pause” on existing projects to better understand the impact of AI-related technologies—rationalizing opportunities, cost to implement, and benefits.
Generative AI requires lots of data to create large language models and improve overall service accuracy. Key factors include what data actually exists, what is potentially available to augment what an organization has, the underlying data quality (accuracy, hygiene, maintainability), the security of the data, and where it is stored. These factors combine to determine how best to implement AI-enhanced services.
Data access—including access to underlying compute platforms and AI models—and issues play a large part in placement decisions as to where AI training and inference loads should be built and run. It’s likely, just like the public cloud for general IT (non-AI-enabled services), that AI will be a hybrid IT model, with services reaching over the network to new data sources and lakes, integrated with existing public and private cloud platforms. This emulates how companies use “hybrid IT” or cloud services today. Moving large amounts of data frequently is costly—both monetarily and the cost of opportunity. Latency is a big factor influencing the time to results and the ability to maintain a competitive advantage in this quickly moving domain.
Wave 4: Autonomous systems
Wave four features a shift towards more autonomous self-learning systems and use cases, with less supervision than the previous waves. The industry has been working on autonomous self-driving cars for decades. We are starting to see use go beyond the pilot stage to real-world implementations and small-scale deployments. As the technology matures, best practices and further technological advancements will drive broader use cases for the industry. This shapes AI much more like its ultimate state—general AI—or general intelligence. It’s still a long way off, but the faster pace of innovation will drive these new use cases with even newer results.
A word of caution
In contrast to the huge amount of potential, AI does introduce many concerns around privacy, disinformation, and faster automation of nefarious and bad-actor use cases. Like any new and advancing technology, there will always be concerns, just like automobiles, rockets, and vaccines. Organizations and governments are best prepared by spending time to understand the potential impacts to learn how to support development while ensuring issues are also addressed.
What the future holds
No matter the wave, AI will revolutionize industries and shape our future. As the significance of AI grows, optimal deployment within data centers requires meticulous consideration of multifaceted factors. Distinctive nuances between training and inference processes, data set access, network latency, and sustainability are paramount. If you are interested in diving deeper into the topic, be sure to download our eBook, “Data centers and the impact of AI,” for a comprehensive look at the intricate relationship between AI and data centers.